Are you looking to establish best practices and ensure your organization’s data quality is up-to-date, accurate, and complete? Well, then this blog post is for you! Knowing how to build an effective data quality management plan can be tricky.
In this post, we will provide practical guidance on creating a plan customized to maximize the value of your data points, so that you can optimize business decisions and capitalize on future opportunities.
After reading our advice you'll have all the resources necessary to start building your successful data quality management Plan!
1. Identify Your Data Quality Goals and Objectives
The first step in creating a data quality management plan is to identify your data quality goals and objectives. It requires a thorough understanding of your organization’s strategic goals, as well as the data needs of different stakeholders.
Your objectives may include ensuring data accuracy, consistency, completeness, and timeliness. You might also aim to improve the usability and accessibility of data across the organization.
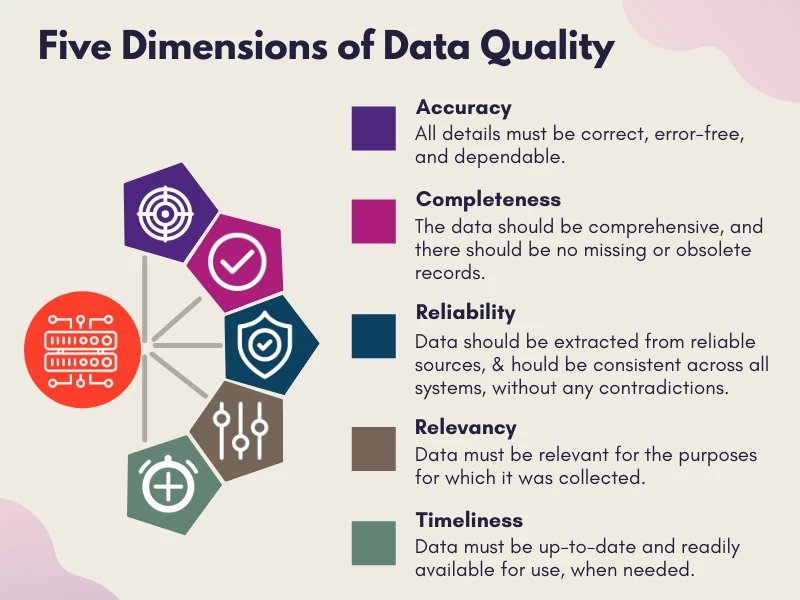
Image Source: Managed Outsource
Every business is unique, and your data quality goals should align with your specific operational needs and strategic vision. Remember, these goals will guide your data quality initiatives and help measure the success of your plan.
2. Create a Data Quality Framework
Once you have identified your data quality goals and objectives, the next critical step is to create a Data Quality Framework. This framework should outline the standards, guidelines, and procedures your organization will follow to maintain high-quality data. It's important that this framework is comprehensive and covers all the vital aspects of data quality, such as data governance, data integration, data validation, and data monitoring.
For instance, the data governance component should identify the roles and responsibilities of each individual involved in managing data quality. The data integration component should outline the methods and tools to be used for integrating data from different sources. The data validation part should specify the techniques for checking and ensuring data accuracy and consistency.
Lastly, the data monitoring component should describe how the quality of data will be tracked over time. This framework will serve as the backbone of your data quality management plan, ensuring that all initiatives align with your stated goals and objectives.
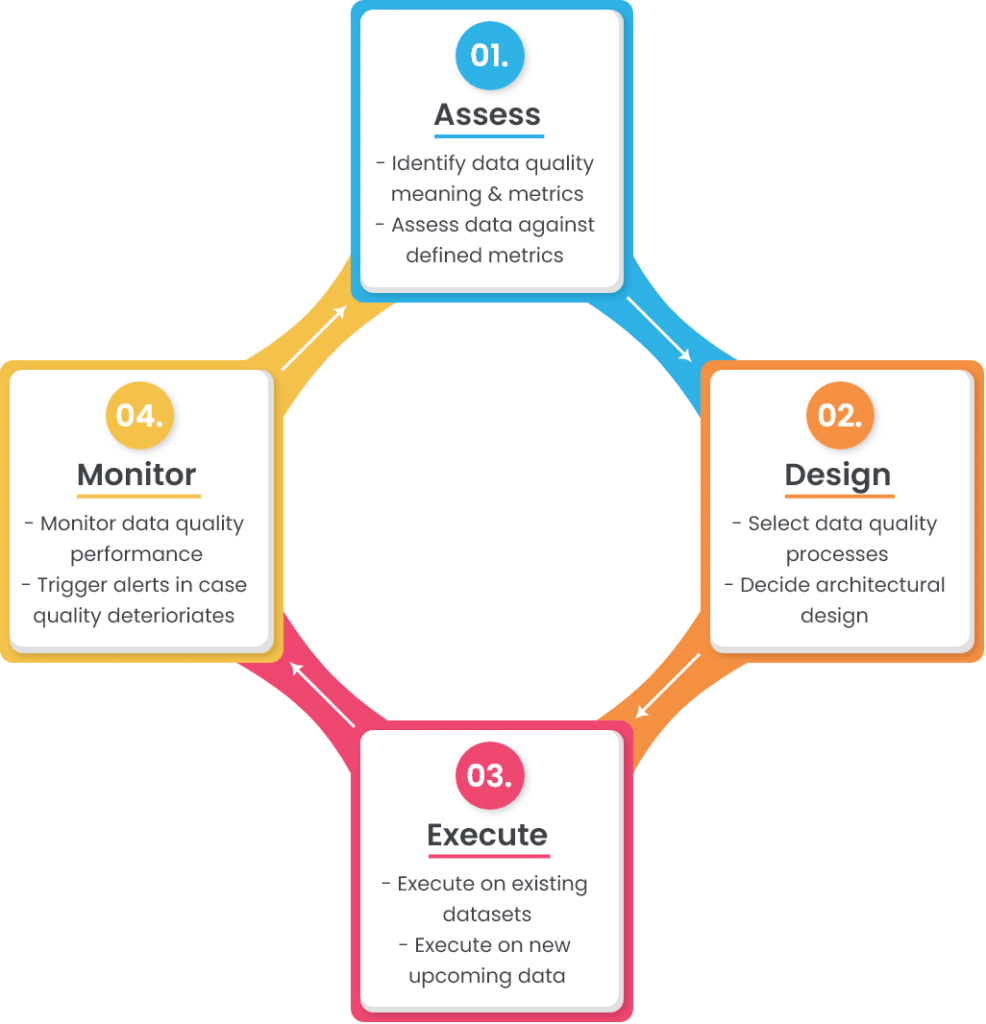
Image Source: DataLadder
3. Assess the Current State of Your Data
The third step in your data quality management plan is to assess the current state of your data. It involves conducting an in-depth data audit to understand the existing quality of your data. You may need to evaluate factors such as data accuracy, uniqueness, completeness, timeliness, and relevance.
This can be achieved through various data profiling techniques, such as checking for inconsistencies in data formats, identifying missing or null values, and finding duplicate records.
Remember, understanding the current state of your data is crucial as it provides a baseline against which you can measure the effectiveness of your data quality improvement initiatives. Additionally, this step may reveal important insights about potential data quality issues that need to be addressed as part of your plan.
4. Develop a Process for Collecting Accurate Data
The next step in your data quality management plan is to establish a robust process for collecting accurate data. It begins with identifying the various data sources your organization utilizes. These might include databases, CRM systems, external vendors, or direct user input. Once you have identified all the data sources, you need to establish a consistent, standardized process for data collection. It involves defining clear data entry protocols and training staff involved in data collection to ensure they understand these protocols.
Data accuracy can also be enhanced by using data validation techniques at the point of data entry. For example, setting rules that require certain fields to be filled in, or that restrict the type of data that can be entered in a given field. Automated data collection methods, such as web scraping or APIs, can also improve accuracy by minimizing the risk of human error.
Furthermore, it's important to maintain an open line of communication with all data providers. Regularly update them on any changes in data collection protocols and provide feedback on the quality of the data they are providing.
It will ensure that they understand the importance of providing accurate data and are fully invested in helping you achieve your data quality goals. By establishing a well-planned process for data collection, you will be laying a strong foundation for high-quality data.
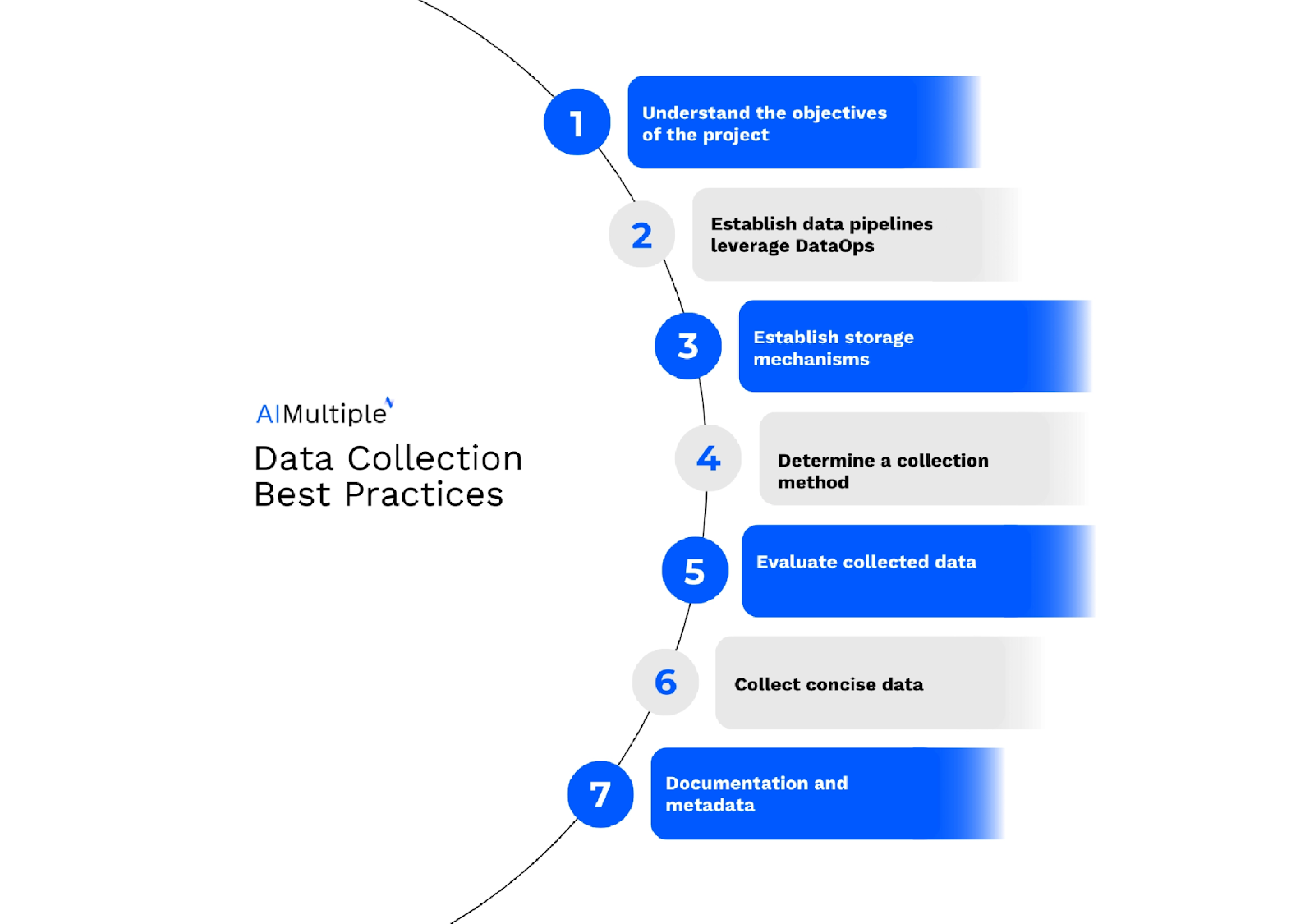
Image Source: AIMultiple
5. Establish Reasonable Standards and Controls for Data Quality
The fifth step in the data quality management plan is to establish reasonable standards and controls for data quality. It involves setting thresholds for data quality metrics, such as accuracy, completeness, uniqueness, timeliness, and consistency. For instance, you might decide that 95% accuracy is acceptable for a particular dataset.
Standards should be realistic, achievable, and aligned with your organization's data quality goals and objectives. They should also be flexible enough to accommodate changes in data volume, data complexity, or business needs. Once you've established your data quality standards, you must implement controls to ensure these standards are consistently met. Controls might include data validation rules, automated data cleaning processes, or regular data quality audits.
For example, you could set up a system that automatically checks new data entries for errors or inconsistencies. Or, you could schedule regular audits to assess the quality of your data and identify any areas for improvement.
By establishing reasonable standards and controls, you'll be able to maintain a high level of data quality and ensure that your data is reliable, accurate, and useful for decision-making.
6. Implement Procedures to Monitor and Maintain Data Quality
The sixth step in your data quality management plan is to implement procedures to continuously monitor and maintain data quality. Ongoing monitoring is essential as it allows for timely detection and resolution of data quality issues. For IT-related data, hardware inventory software provides real-time tracking and reporting on asset statuses, enabling your team to proactively manage and maintain accurate data. You can use data monitoring tools that provide real-time reports, dashboards, and alerts about potential data inconsistencies or errors.
One effective method is to establish Key Performance Indicators (KPIs) that align with your data quality goals. These KPIs should be regularly reviewed to ensure your data meets the set quality standards. For example, if one of your goals is data completeness, a relevant KPI might be the percentage of incomplete records in your database.
In addition to monitoring, regular maintenance is equally important. It could involve routine data cleaning tasks, such as removing duplicate records, filling in missing values, or correcting inaccuracies. Automated data-cleaning software can be employed to streamline these processes. Remember, maintenance is not a one-time task but a continual effort to preserve the integrity and relevance of your data.

Image Source: Techtarget
Lastly, it's important to review and update your data quality management plan periodically. As your business evolves, so will your data needs and quality goals. Regular reviews will ensure your plan remains effective and aligned with your organization's strategic objectives. By implementing rigorous monitoring and maintenance procedures, you will ensure the ongoing reliability and value of your data.
7. Use Automation to Streamline the Process and Improve Efficiency
In today's fast-paced digital environment, manually managing data quality can be time-consuming and prone to errors. Hence, one crucial step in your data quality management plan should be to harness the power of automation to streamline processes and improve efficiency. Automation can be employed in various aspects of data quality management, from data collection and validation to monitoring and maintenance.
Automated data collection tools, such as APIs or web scraping technologies, can significantly reduce the time and effort required to gather data and minimize the risk of human error. Similarly, automated data validation can help to promptly identify and rectify errors or inconsistencies in data.
Automated data cleaning and enrichment tools can help you maintain the quality of your data by routinely performing tasks like removing duplicates, filling in missing values, and correcting inaccuracies. This not only ensures the accuracy and completeness of your data but also frees up valuable time and resources that can be better deployed elsewhere.
Finally, automated data monitoring tools can provide real-time insights into your data quality, alerting you to potential issues before they become significant problems. These tools can generate dashboards and reports that track your key data quality metrics and KPIs, enabling you to make informed decisions and continuously improve your data quality.
Leveraging automation in your data quality management plan will enhance data accuracy, consistency, and timeliness and lead to significant cost and time savings. Thus, it is a critical component to ensure an efficient, effective, and sustainable data quality management process.
8. Analyze Performance Metrics to Measure Success
The final step in your data quality management plan is to analyze performance metrics to gauge the success of your data quality initiatives. These metrics should be tied to your data quality goals and objectives defined earlier in the plan. Common metrics include data accuracy, completeness, uniqueness, consistency, and timeliness.
For instance, if one of your goals is to improve data accuracy, you might measure the percentage of records containing errors. A drop in this percentage over time would indicate progress toward your goal. Similarly, if your objective is to enhance data completeness, you could track the number of incomplete records. A declining trend in this metric would demonstrate success in achieving your objective.
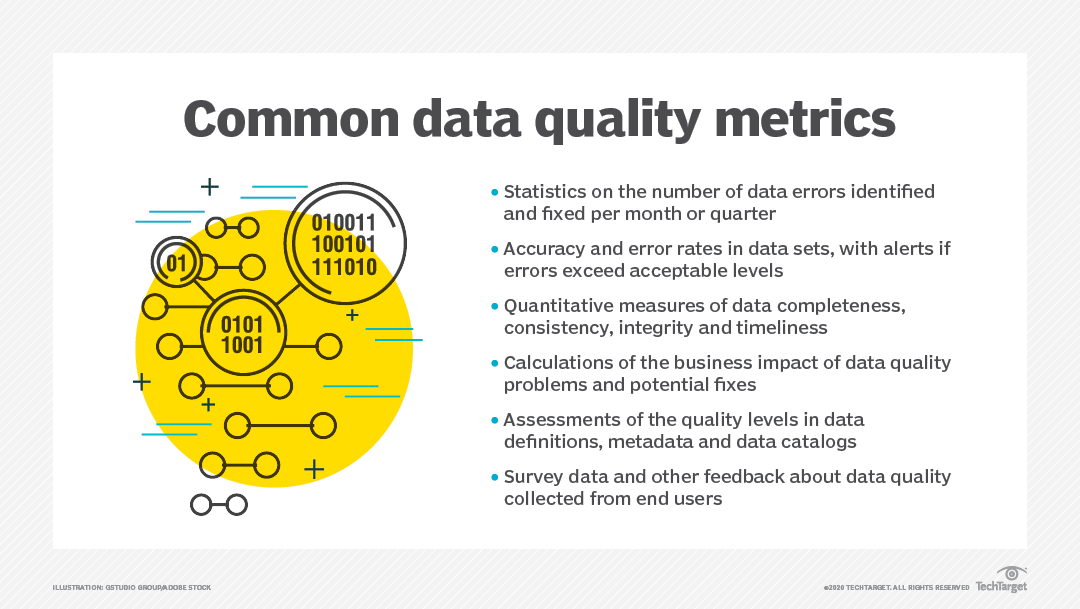
Image Source: TechTarget
In addition to these quantitative measurements, qualitative assessments can also provide valuable insights. Feedback from data users regarding the usefulness and accessibility of data can help you understand if your initiatives are delivering the expected benefits.
Use a data quality dashboard to visualize these metrics for easy analysis and interpretation. Regularly review these performance metrics and compare them against your benchmarks to identify areas of success and opportunities for improvement. Remember, the objective of analyzing these metrics is not just to measure performance, but to gain insights that can drive continuous improvement in your data quality management efforts.
Successfully managing data quality is an ongoing process that requires continuous monitoring, evaluation, and adjustment. But with a structured data quality management plan in place, you'll be well-equipped to ensure the integrity, reliability, and value of your data.
The white label dashboard software by DashClicks is a powerful tool that can significantly assist in your data quality management plan. It offers a central location to monitor and manage all your data, providing real-time insights and analytics that can drive informed decision-making.
For instance, the software can help automate data collection. With its ability to integrate with various data sources, it ensures that data gathering is consistent and error-free. Additionally, the software offers automated data validation features that can check for inconsistencies and errors as data is being collected, ensuring its accuracy from the get-go.
Moreover, client dashboard allows for real-time monitoring of your data. You can define your KPIs and track them on the dashboard, giving a visual representation of your data quality. You can set alerts to be notified when data quality metrics fall below your set standards, allowing for immediate corrective action.
White label reporting dashboard also offers automation for routine data cleaning tasks. It can detect and remove duplicates, fill in missing values, and correct inaccuracies, ensuring the maintenance of high-quality data.
Finally, Dashclicks can assist in analyzing performance metrics to measure the success of your data quality initiatives. Its customizable dashboard allows you to visualize and track your crucial data quality metrics over time, making it easier to identify trends, successes, and areas for improvement. With Dashclicks’ white label dashboard, managing data quality becomes a streamlined and efficient process.
In Conclusion
Developing an effective data quality framework should be a primary goal for organizations seeking to make better business decisions and create value from their data. By defining data objectives, assessing the current state of your data, implementing sound processes, standards, controls, and automation, and measuring performance analytics you can ensure high-quality data that can impact profitability.
As the importance of high-quality data continues to grow in relevance, it is worthwhile for organizations to invest time and resources towards building a robust data quality framework that leads to improved decision-making. Executing these steps should set your organization up for success as you strive to become a leader in leveraging quality information systems.
So now put this advice into practice right away—start creating plans for a more secure and reliable future with strong data quality protocols!
